10 Data Mistakes Costing Your Fashion Brand Millions
Fashion brands lose millions each year due to poor data practices, from ignoring customer sentiment to misreading product performance. Learn the 10 most common data mistakes and how to fix them to turn insight into impact.
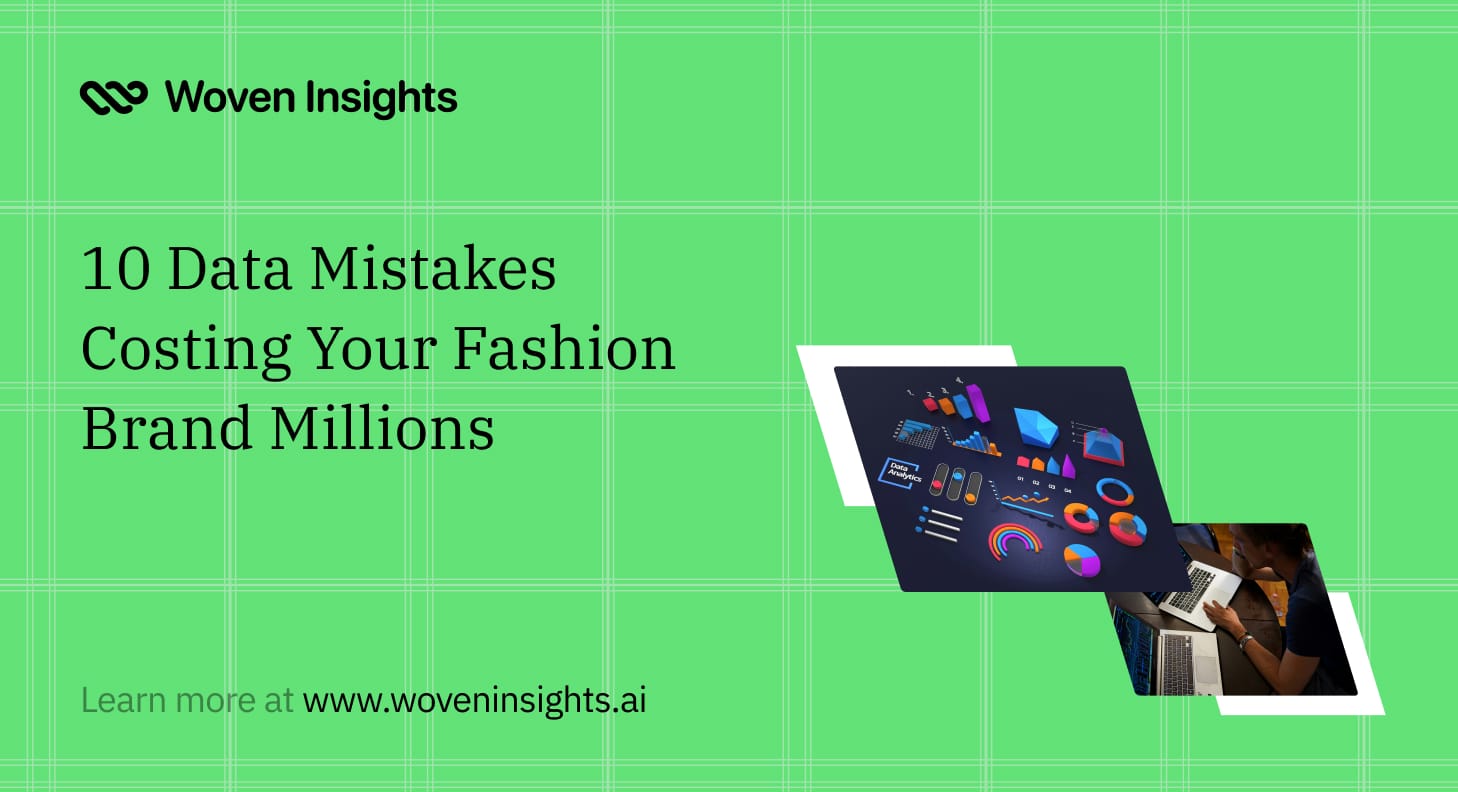
In today’s data-driven world, fashion brands can no longer afford to make blind decisions. Data holds the key to understanding consumers, optimizing operations, and outperforming competitors. Yet, many fashion brands unknowingly sabotage themselves with poor data practices, leading to missed revenue, wasted marketing budgets, and costly inventory mistakes. Below are 10 costly data mistakes that could be draining millions from your brand And how to fix them
1. Ignoring Customer Sentiment
Fashion isn’t just about selling products — it’s about selling feelings. Yet, many brands focus solely on sales data while ignoring what customers are actually saying. Reviews, social media comments, and customer feedback contain invaluable insights about fit, quality, and style preferences. Overlooking sentiment analysis leaves brands blind to evolving customer expectations, which can result in declining loyalty and lost sales.
The Fix:
Adopt sentiment analysis tools that mine customer feedback for recurring themes around fit, quality, style, and more. Incorporate these insights into product development and marketing messaging.
2. Relying on Gut Feeling Over Data
Fashion thrives on creativity, but decisions based purely on instincts or trends without supporting data are risky. Whether it’s planning assortments, setting prices, or launching new collections, decisions should be backed by data from consumer behavior, market trends, and past performance to minimize risks and maximize returns.
The Fix:
Combine creative intuition with real-time data on consumer preferences, past product performance, and market dynamics. Make data a required input in product lifecycle decisions.
3. Poor Quality or Incomplete Data
Incomplete, inconsistent, or outdated data leads to poor decision-making. For example, missing product attributes, inaccurate sizing data, or outdated customer profiles can result in stockouts, overproduction, or ineffective marketing. Brands without a data quality management process are essentially making decisions with blurred vision.
The Fix:
Implement a data governance framework. Regularly clean, validate, and enrich your data, especially around product attributes, inventory, and customer profiles.
4. Neglecting Competitor Intelligence
Many brands only focus inward, ignoring what competitors are doing. Competitor data reveals pricing strategies, assortment gaps, emerging trends, and customer perceptions of rival products. Without this, brands miss out on opportunities to outperform competitors and capture market share.
The Fix:
Set up automated competitor monitoring tools to track assortments, pricing changes, customer ratings, and visual trends. Use this intel to inform your assortment, pricing, and marketing strategy.
5. Failing to Connect Online and Offline Data
With the rise of omnichannel shopping, brands often fail to integrate data from physical stores, e-commerce platforms, and social channels. Disconnected data leads to poor inventory allocation, fragmented customer experiences, and missed revenue opportunities. A unified view is essential for accurate demand forecasting and customer insights.
The Fix:
Adopt an omnichannel analytics platform that unifies data across all touchpoints. This provides a single customer view and better inventory forecasting and personalization.
6. Overlooking Regional and Cultural Differences
Global brands sometimes assume that what works in one market will work everywhere. Ignoring regional trends, sizing variations, cultural preferences, and local competitors can result in poorly performing collections. Data should always be segmented by region to tailor offerings and messaging appropriately.
The Fix:
Segment your data by geography and demographic. Tailor product offerings, marketing, and even sizing to local preferences and behaviors.
7. Inadequate Product Performance Tracking
Not all products contribute equally to a brand’s success. Without detailed product performance analytics — including sell-through rates, return reasons, and customer reviews — brands risk continuing to invest in underperforming styles while ignoring star products. This leads to wasted production costs and lost profit potential.
The Fix:
Track a richer set of metrics — including return reasons, review sentiment, margin contribution, and repeat purchase rates. Use this to refine future collections and drop under-performers quickly.
8. Misinterpreting Data or Drawing Superficial Insights
Having data is not enough — interpreting it correctly is key. Fashion brands sometimes rely on vanity metrics (e.g., social media likes) without connecting them to revenue-impacting KPIs like conversion rates, average order value, or customer retention. Shallow analysis leads to misguided strategies.
The Fix:
Train teams to dig deeper. Link marketing metrics to sales, engagement to retention, and product views to conversion. Invest in dashboards that tie insights to revenue.
9. Underestimating the Power of Visual Data
In fashion, visuals matter. Yet, brands often neglect to analyze visual trends in product images, influencer content, or competitor assortments. Visual AI can detect emerging patterns in color, style, and design elements. Ignoring visual data analysis means missing the next big trend before it hits mainstream.
The Fix:
Use visual AI to analyze trending colors, patterns, silhouettes, and styling cues. Integrate these insights into design and merchandising strategies.
10. Lack of Continuous Data-Driven Innovation
Fashion is fast, and consumer preferences change constantly. Brands that don’t regularly update their data strategies, monitor performance, or experiment with new analytics tools risk falling behind. Continuous improvement fueled by real-time data is no longer optional — it’s a competitive necessity.
The Fix:
Make data central to your culture. Run regular tests, evaluate new data tools, and use insights to drive continuous iteration across products, marketing, and operations.
Conclusion
Data mistakes aren’t just technical slip-ups, they’re strategic blind spots. In the fast-moving fashion world, the difference between winning and falling behind often comes down to how effectively you use data. Clean it, connect it, and act on it, or risk paying the price.