Optimize Inventory with Data-Driven Retail Forecasting
Discover how data-driven retail forecasting transforms inventory management. Learn actionable strategies and insights using advanced analytics and AI tools like WovenInsights to ensure the right products are always on the shelf.
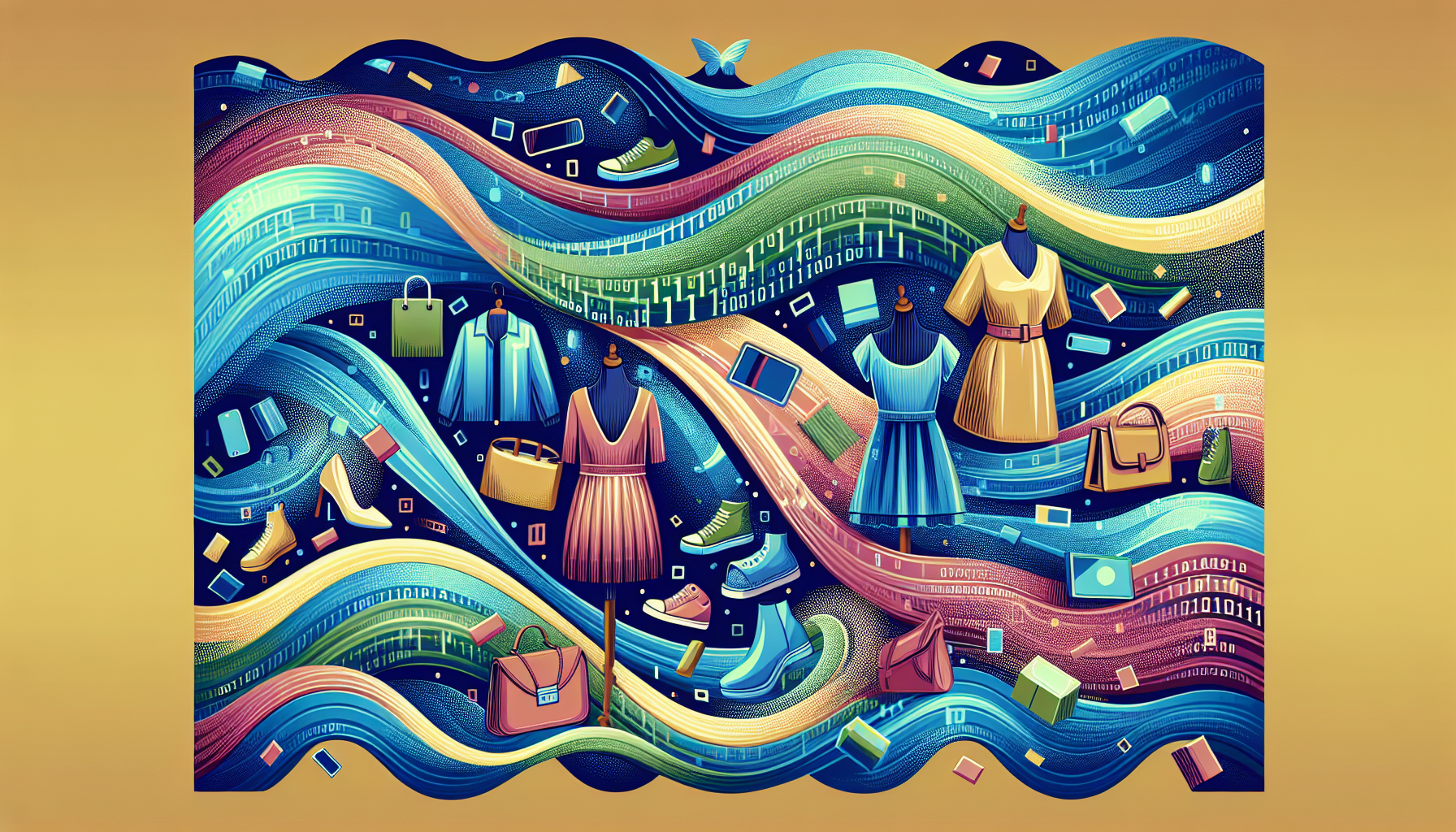
Introduction
In the world of retail, efficiently managing inventory is more critical than ever. Retailers are increasingly turning to advanced data analytics to predict demand, avoid overstocking, and minimize stock-outs. A data-driven approach not only streamlines inventory management but also enhances overall profitability. With powerful business intelligence platforms like Woveninsights, fashion brands and retailers have access to actionable insights that can transform their supply chain and inventory practices.
Table of Contents
- The Evolution of Inventory Forecasting
- Understanding Data-Driven Retail Forecasting
- Leveraging Advanced Technologies
- Practical Strategies to Optimize Inventory
- Implementing Data-Driven Inventory Solutions
- Case Study: Transforming Inventory in Fashion Retail
- Emerging Trends in Demand Forecasting
- Conclusion
The Evolution of Inventory Forecasting
The landscape of inventory management has shifted dramatically over the years. Traditional methods, based largely on historical experience and gut instinct, have given way to predictive analytics and smart forecasting techniques. This transformation has been driven by the availability of granular data and the development of advanced analytical tools.
Retailers now understand that responding to fluctuations in consumer demand requires more than just reactive inventory management. Data-driven forecasting enables a proactive approach, aligning stock levels with emerging trends and customer preferences. This methodology not only reduces excess inventory costs but also helps maintain customer satisfaction by ensuring that popular products are always in stock.
Understanding Data-Driven Retail Forecasting
Retail forecasting involves the prediction of future consumer demand using historical data, seasonality patterns, and emerging market trends. This process is at the heart of a robust inventory strategy and includes several key components:
- Historical Sales Analysis: Evaluating past sales data to identify recurring patterns and trends.
- Predictive Analytics: Using statistical models and machine learning to forecast future demand accurately.
- Consumer Sentiment Analysis: Gauging customer feedback to assess the market perception of products.
- Competitive Benchmarking: Analyzing competitor performance to adjust stock levels and pricing strategies.
Advanced forecasting involves blending these elements to create a holistic view of the market. For fashion retailers, where trends can shift quickly, having real-time data on customer sentiment and market trends is invaluable.
Leveraging Advanced Technologies
Data-driven retail forecasting is made possible by a combination of modern technologies:
Artificial Intelligence and Machine Learning
AI and machine learning models process vast amounts of data to identify patterns that human analysis might overlook. These models excel at recognizing seasonal fluctuations, regional variations, and sudden market shifts. Platforms like WovenInsights utilize AI-powered recommendations to fine-tune forecasts, ensuring that inventory levels meet consumer demand without generating unnecessary waste.
Cloud-Based Analytics
Cloud platforms enable retailers to collect, store, and analyze data in real-time. This centralized approach ensures that decision-makers have access to the latest information no matter where they are. With continuously updated dashboards, fashion retailers can monitor key performance indicators (KPIs) such as inventory turnover rate, lead times, and stock-out incidents.
Internet of Things (IoT) and Smart Sensors
IoT devices—like RFID tags and smart shelf sensors—offer real-time insights into inventory levels. These tools automatically track product movement, alerting managers when stock reaches critical thresholds. When integrated with AI-driven platforms, this real-time data supports dynamic forecasting models that adjust replenishment schedules instantly.
Data Visualization and Reporting
Effective forecasting isn’t just about collecting data; it’s about understanding it. Data visualization tools convert complex analytics into clear, actionable insights. Retail managers can quickly identify trends and bottlenecks, making informed decisions on stock replenishment and promotional strategies.
Practical Strategies to Optimize Inventory
When it comes to inventory management, practical strategies backed by data can yield significant improvements. Here are several actionable strategies that fashion retailers can adopt:
1. Segment Your Inventory
Different products have different demand patterns. Segment inventory into fast-moving, moderate, and slow-moving categories. This segmentation allows for tailored forecasting and replenishment schedules. Advanced analytics can condition forecasts for each product category, ensuring precision in inventory levels.
2. Monitor Seasonal Trends
Seasonal fluctuations are inevitable in retail, particularly in the fashion industry. Use historical sales data and market trend analysis to capture the impact of seasonal demand spikes and troughs. Integrating social media sentiment and trend analysis helps predict when consumers are likely to show interest in certain styles or collections.
3. Implement Real-Time Data Tracking
Adopt technology that offers real-time monitoring of inventory. Real-time dashboards and alerts allow retail managers to respond swiftly to supply chain disruptions or unexpected demand surges. This approach minimizes the risk of having too much or too little inventory.
4. Leverage AI-Powered Recommendations
AI tools can analyze historical data, consumer behavior, and market trends to provide actionable recommendations regarding stock levels and pricing adjustments. By relying on these predictive recommendations, retailers optimize inventory without relying solely on guesswork.
5. Foster Cross-Departmental Collaboration
Inventory management is not a single-department function. Encourage collaboration between marketing, sales, supply chain, and customer service teams. A unified approach ensures that all stakeholders are on the same page, contributing their perspectives and data insights for more robust forecasting.
Implementing Data-Driven Inventory Solutions
For many retailers, transitioning to a data-driven approach can seem daunting. However, a step-by-step strategy can simplify the process.
Step 1: Data Collection and Cleansing
Before any analysis can occur, retailers need accurate and comprehensive data. Collect data from various touchpoints (POS systems, online sales, customer reviews) and ensure it undergoes thorough cleansing. High-quality data forms the backbone of reliable forecasts.
Step 2: Historical Sales and Trend Analysis
Analyze past sales records to identify repeatable trends. Tools that provide detailed visualizations of sales performance for individual stock-keeping units (SKUs) can be particularly useful here. With historical data in hand, retailers can better understand cyclical patterns and prepare for seasonal changes.
Step 3: Apply Advanced Forecasting Techniques
With a robust dataset, apply predictive analytics models. Machine learning algorithms help in identifying hidden trends and correlations in the sales data. The predictive insights derived can then be used to adjust order quantities and timing.
Step 4: Utilize Dynamic Reporting Tools
Deploy dashboard and reporting tools that offer real-time visibility into inventory performance. These reports should be accessible to key stakeholders across departments. Dynamic reporting enables quick adjustments to replenishment schedules and ultimately reduces both overstock and stock-out scenarios.
Step 5: Continuous Improvement and Monitoring
Adopting a data-driven approach is an ongoing process. Continuously monitor KPIs such as inventory turnover, stock-out frequency, and lead times. Regularly update forecasting models with new data to refine their accuracy over time.
For a seamless experience, platforms like Woveninsights consolidate these functions into a single, cloud-based solution. They provide tools ranging from customer sentiment analysis to competitor benchmarking, all of which are critical to creating a comprehensive forecasting model.
Case Study: Transforming Inventory in Fashion Retail
Consider the example of a mid-sized fashion retailer facing issues with seasonal overstock. By integrating a data-driven forecasting system, the retailer was able to pinpoint which products sold well during specific periods. The system monitored not only historical sales but also real-time customer sentiment and emerging market trends. As a result:
- Stockouts were reduced by 25%: Real-time data allowed for swift adjustments to reorder levels.
- Excess inventory dropped significantly: Predictive analytics helped avoid overordering by aligning purchase orders with actual demand.
- Collaborative decision-making improved: Departments across the organization shared insights via dynamic dashboards, ensuring everyone was in tune with market conditions.
This transformation was made possible by adopting a platform that offers in-depth data visualization and actionable insights—core features of WovenInsights.
Emerging Trends in Demand Forecasting
The dynamics of demand forecasting are continually evolving. Several emerging trends are set to shape the future of inventory management:
Integration of Social Media Sentiment Analysis
Real-time sentiment analysis from social media platforms provides valuable cues on upcoming trends. Retailers who integrate this data into their forecasting models can anticipate changes in consumer behavior with greater accuracy.
Enhanced Predictive Modeling with AI
Continuous improvements in AI and machine learning mean that forecasting models are becoming increasingly sophisticated. Future systems will likely offer even more granular insights, adapting predictions in real time as new data is fed into the system.
Omnichannel Inventory Visibility
As retailers diversify their channels—from physical stores to online marketplaces—maintaining a unified view of inventory becomes crucial. Cloud-based business intelligence platforms ensure seamless integration across channels, allowing for holistic inventory management.
Sustainable Inventory Practices
Data-driven forecasting not only improves efficiency but also contributes to sustainability. By aligning inventory levels closely with actual demand, retailers reduce waste and optimize resource use. This alignment is essential for brands committed to ethical and sustainable practices.
Conclusion
Data-driven retail forecasting has redefined inventory management. By harnessing advanced analytics, AI-powered recommendations, and real-time insights, retailers can successfully navigate the complexities of modern demand forecasting. Whether it’s segmenting inventory or integrating real-time data from IoT devices, the actionable insights provided by platforms like WovenInsights empower retailers to make informed, strategic decisions.
By leveraging these technologies, fashion businesses can not only optimize their inventory but also enhance overall operational efficiency and customer satisfaction. As forecasting models continue to evolve, those who invest in data-driven solutions today will be better positioned to lead in the marketplace tomorrow.
Are you ready to optimize your inventory with data-driven forecasting? Discover how WovenInsights can transform your retail operations by visiting our website and exploring our innovative tools. Embrace the future of inventory management, reduce waste, and drive profitability with precision.
About WovenInsights
WovenInsights is a comprehensive market analytics solution that provides fashion brands with real-time access to retail market and consumer insights, sourced from over 70 million real shoppers and 20 million analyzed fashion products. Our platform helps brands track market trends, assess competitor performance, and refine product strategies with precision.
WovenInsights provides you with all the actionable data you need to create fashion products that are truly market-ready and consumer-aligned.
Click on the Book a demo button below to get started today.