Predict Fashion Demand: Data-Driven Techniques That Work
Discover powerful, data-driven demand forecasting techniques that elevate fashion brands. Learn how to predict trends, reduce inventory risks, and leverage cutting-edge platforms like WovenInsights for maximum market impact.
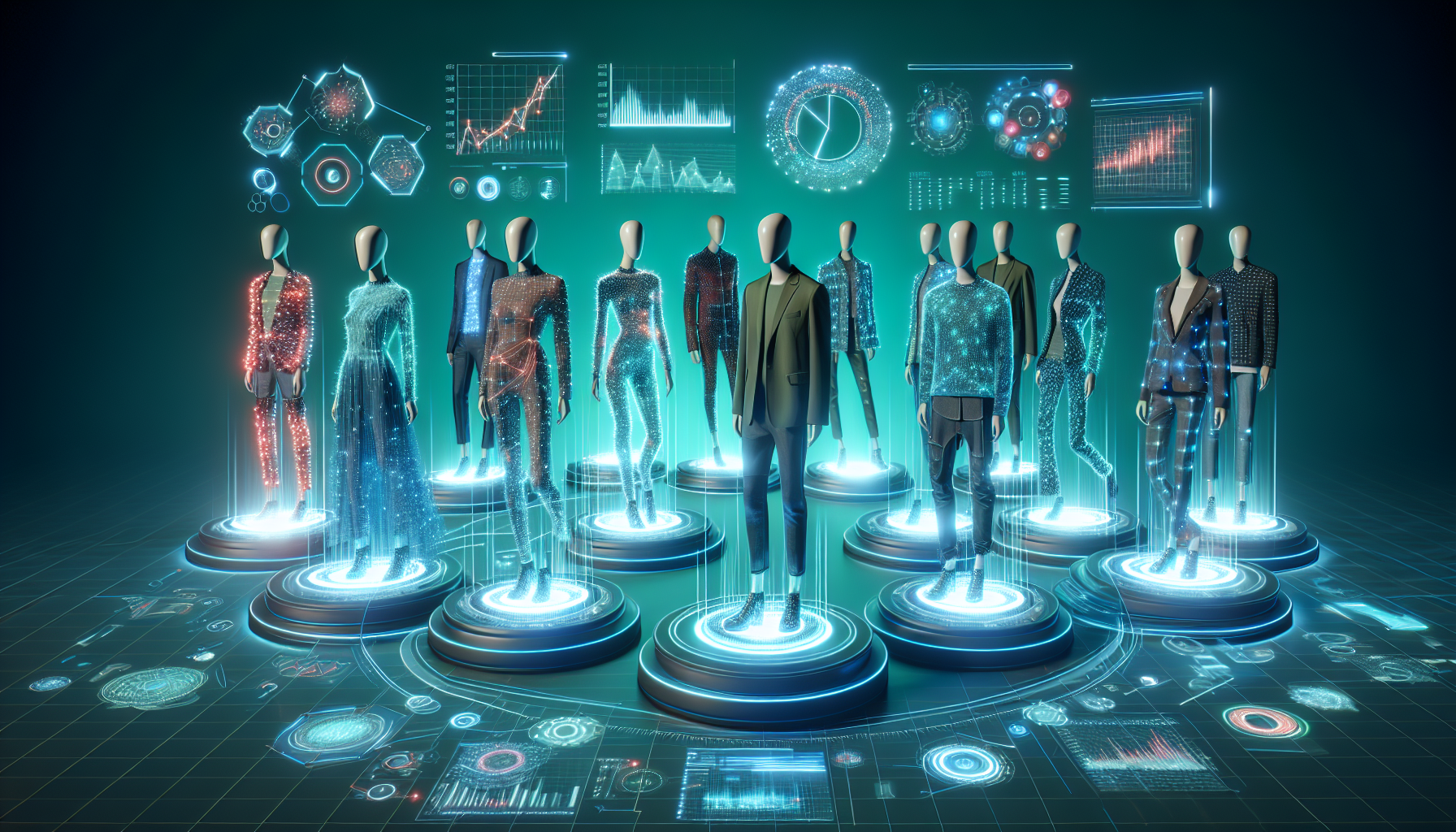
Introduction
Forecasting demand in fashion has always been a balancing act between art and science. With trends changing faster than ever, relying solely on intuition or historical trends can lead to missed opportunities or overstocked inventory. Data-driven demand forecasting transforms guesswork into actionable insights, helping businesses keep pace with evolving consumer preferences. This article explores powerful techniques, real-world examples, and essential tools for predicting fashion demand. It also highlights how platforms like Woven Insights enable brands to stay agile in a rapidly changing market.
Table of Contents
- Why Demand Forecasting Matters in Fashion
- Key Components of a Successful Forecasting Strategy
- Predictive Models & Techniques
- Building an Actionable Forecasting Plan
- Common Pitfalls and How to Avoid Them
- Real-World Examples
- Actionable Tips for Refining Your Demand Forecasting Process
- Conclusion
Why Demand Forecasting Matters in Fashion
Fashion is notorious for rapidly changing trends, unique consumer preferences, and strong social influences. A single must-have season can morph into a global phenomenon in mere weeks, leaving retailers scrambling to secure enough stock, or worse, stuck with excess inventory if the hype dwindles. With demand forecasting, you can:
- Optimize Inventory Levels: Minimize overstock and understock situations, reducing markdowns and cutting storage costs.
- Stay Ahead of Trends: Use data to anticipate upcoming styles and color palettes, so your brand appears innovative.
- Enhance Profitability: Forecasting helps decide the right product mix, pricing strategies, and marketing efforts.
- Improve Customer Satisfaction: Maintaining steady stock of popular items encourages loyalty and repeat purchases.
According to a Harvard Business Review study, brands using advanced customer analytics can outperform peers by 2–3 times in sales, margins, and profitability. Predictive analytics and robust intelligence solutions give fashion businesses a keen edge in aligning products with consumer demand.
Key Components of a Successful Forecasting Strategy
Data Collection & Integration
Your forecasting success depends on the quality and diversity of data you feed into your models. Relevant sources include:
- Sales History: Past performance, seasonal spikes, and historical bestsellers.
- Customer Feedback & Reviews: Sentiment analysis tools reveal emerging issues or product dissatisfaction.
- E-commerce and Foot Traffic Data: Website clicks, conversion rates, in-store sensor data, and heatmaps.
- Social Media Metrics: Hashtags, mentions, and trending topics signal early interest or declining buzz.
- Macro-Economic Indicators: Consumer spending habits often shift with economic changes.
Efficient aggregation of raw data is crucial. Platforms like Woven Insights streamline data integration, generating a single source of truth for smooth collaboration across departments.
Market Trend Analysis
Anticipating style direction, color palettes, and customer lifestyles is vital for maintaining a competitive edge. Common methods:
- Trend Observatories: Dedicated trend analysis services (e.g., WGSN) track consumer preferences.
- Runway Monitoring: Catwalks serve as bellwethers, and certain highlights can quickly go mainstream.
- Influencer Impact: Fashion influencers often spark viral styles, creating instant market demand.
- Seasonality Considerations: Factor in climate changes and cultural events that influence clothing choices.
Using historical data combined with real-time trend data keeps your brand poised for timely product launches.
Consumer Sentiment Analysis
Consumers increasingly share opinions on social media, review platforms, and community forums. Understanding these perspectives allows brands to:
- Identify Emerging Pain Points: Detect dissatisfaction with a fabric or design approach before it grows.
- Refine Marketing Messages: Focus on what truly resonates with consumers, whether it’s sustainability or unique designs.
- Adopt Continuous Improvement: Gather input and apply insights to upcoming collections.
Tools like Woven Insights’ Customer Sentiment Analysis provide AI-driven breakdowns of consumer emotion, star ratings, and frequently used keywords essential for spotting micro-trends quickly.
Competitive Benchmarking
Demand forecasting isn’t limited to your own brand. Keeping tabs on competitor moves, product launches, and pricing offers a reality check for your predictions. Consider:
- Pricing Strategies: If competitors go on sale early, it may sway your promotional calendar.
- Product Mix Overlaps: Understanding your shared audience can help you refine selections.
- Brand Sentiment: Compare consumer perceptions and social chatter to highlight gaps in your product line.
Woven Insights includes competitor benchmarking so you can proactively adjust your demand forecasts, ensuring your brand is never caught off-guard.
Predictive Models & Techniques
Time Series Analysis
This classic approach uses historical sales data to predict future demand. Seasonal ARIMA (Autoregressive Integrated Moving Average) is a popular model for capturing seasonal, trend, and cyclical components of fashion data:
- Strengths: Straightforward with relatively low data requirements
- Limits: Less effective when consumer behavior changes drastically, such as abrupt shifts fueled by social media
Time series analysis is often a solid foundation but pairing it with machine learning captures additional consumer behaviors.
Machine Learning Approaches
Machine learning integrates multiple variables—social media mentions, competitor pricing, brand sentiment, local events—to provide dynamic forecasts. Random Forest, Gradient Boosting, and Neural Networks can handle high-dimensional data sets. This approach:
- Learns Complex Relationships: Gleans insights from interactions between variables.
- Updates Continuously: Learns from new data in near-real time.
- Requires Rigorous Validation: Overfitting can occur if models aren’t properly monitored.
Sources such as Intellias highlight how machine learning can refine sales predictions for new product launches, especially during the initial weeks.
AI-Driven Insights
Artificial intelligence takes machine learning a step further. AI-powered solutions can:
- Process High Volumes of Data: Handle millions of consumer reviews, social media posts, and competitor stats.
- Uncover Hidden Patterns: Recognize micro-trends, niche customer segments, or subtle emotional cues.
- Generate Automated Recommendations: Update retailers on potential hot products or underperforming categories.
Woven Insights harnesses AI to offer real-time demand insights, bridging the gap between raw data and strategic decisions.
Building an Actionable Forecasting Plan
Goal Setting & KPI Alignment
Any forecasting strategy must align with overarching business goals:
- Revenue Targets: If your brand aims for a 20% increase in a quarter, plan inventory and marketing accordingly.
- Sell-Through Rates: Ideal for gauging how efficiently products move through your channels.
- Profit Margins: Over-forecasting leads to markdowns, under-forecasting leaves money on the table.
Be realistic in your KPI definitions. Embed them in your planning so each department can work cohesively toward shared objectives.
Tool Selection & Data Visualization
Organizing your forecasts, analyzing trends, and sharing insights with stakeholders all hinge on user-friendly dashboards. Key considerations:
- Usability: Ensure teams can easily interpret complex data.
- Real-Time Updates: Forecasts must refresh with every new dataset, especially during high-demand seasons.
- Collaborative Features: Finance, design, and marketing teams should be able to comment, revise, and adapt forecasts collectively.
Woven Insights offers data visualization modules, allowing fashion professionals to interpret market signals clearly and share findings seamlessly.
Forecast Monitoring & Adjustment
A forecast is only as good as your ability to revise it:
- Track Actual Performance: Monitor daily or weekly sales, inventory turnover, and conversion rates.
- Identify Deviations: If an item sells out faster than expected, adjust future purchase orders.
- Incorporate Feedback Loops: Encourage store managers and e-commerce teams to share frontline intel.
- Evaluate Model Accuracy: Compare predicted vs. actual performance, refining forecasting models over time.
Consistent monitoring ensures your forecasting remains an ongoing feedback mechanism rather than a one-off exercise.
Common Pitfalls and How to Avoid Them
Over-Reliance on Historical Trends
Historical data offers valuable context. However, ignoring present-day consumer behaviors, new influencers, or unexpected events risks outdated forecasts.
How to Avoid: Pair historical data with real-time market intelligence from social media, competitor analysis, and sentiment tracking.
Ignoring Market Disruptions
Pandemics, sudden viral trends, supply chain breakdowns global disruptions can invalidate your best-laid plans.
How to Avoid: Build contingency forecasts that account for worst-case and best-case scenarios.
Underestimating the Importance of Inventory Allocation
Forecasting total demand is only half the puzzle. Getting the right stock to the right channels is vital.
How to Avoid: Use location-based demand analyses, factoring in store-specific data and demographic differences.
Real-World Examples
E-Commerce Resilience
A mid-sized online boutique selling eco-friendly athleisure gear struggled with constant stockouts. By employing AI-driven analysis and time series forecasting, the brand:
- Analyzed Social Media Mentions: Spotted rising interest in pastel-toned leggings.
- Refined Inventory Reorders: Ensured an ample supply of these in-demand shades.
- Boosted Sell-Through Rates: Maintained smaller surplus while meeting the surge in orders.
Revenue soared, and markdowns all but vanished. This shift was fueled by continuous data monitoring rather than guesswork.
Brick-and-Mortar Optimization
A global footwear retailer implemented advanced demand forecasting in stores across 10 major cities:
- Localized Demand Forecasts: Factored in regional styles and climates.
- Dynamic Pricing Tests: Adjusted prices in mid-season for select locations with oversupply.
- Customer Segmentation: Gauged which cohorts preferred bright sneakers versus understated neutrals.
By the end of the quarter, stores reduced unsold inventory by 15% and improved profit margins significantly.
Woven Insights Use Cases
Several fashion brands leverage Woven Insights to:
- Aggregate High-Volume Data Sources: Consolidate everything from e-commerce cart abandonment rates to supply chain lead times.
- Detect Hot or Underperforming Items: AI-powered modules scan for anomalies in real time.
- Drive Collaborative Planning: Cross-functional teams utilize a single dashboard, accelerating decision-making.
- Optimize Marketing Campaigns: Marketers identify winning messaging strategies and channels by analyzing consumer engagement data.
- Predict Future Trends & Pricing: Intelligent forecasting tools suggest timely inventory adjustments and promotional windows.
The result? Brands gain a data-backed roadmap to maximize revenue, minimize waste, and cultivate brand loyalty.
Actionable Tips for Refining Your Demand Forecasting Process
Leverage Social Media and Reviews
Fashion thrives on social proof. User-generated content, crowd-sourced reviews, and influencer mentions paint a clear picture of consumer sentiment:
- Keyword Monitoring: Track how consumers describe your brand or products.
- Hashtag Analysis: Identify emergent subcultures or style movements.
- Sentiment Trends: Shift marketing messages when feedback indicates a potential misalignment.
Adopt Dynamic Pricing Strategies
Adjusting prices in real time—or at least more frequently—keeps your brand competitive and helps clear slow-moving inventory. Follow the lead of major online retailers who tweak prices daily based on:
- Demand Levels
- Competitor Pricing
- Inventory Positions
When done ethically and transparently, dynamic pricing can significantly boost both margins and customer satisfaction.
Plan for Sustainability and Ethical Practices
As consumer sentiment evolves, sustainability and ethical sourcing rise in priority. Considering these elements within your demand forecasts makes sense:
- Incorporate Eco-Friendly Materials: Factor in emerging green trends and cost fluctuations for sustainable fabrics.
- Track Consumer Perception: Browns, beiges, and “natural” designs may cycle more frequently in an ethically conscious consumer base.
- Plan for Extended Lifecycles: Embrace the slow-fashion model by forecasting timeless, high-quality pieces that last longer.
In addition, Woven Insights can help brands measure the effectiveness of sustainability-related marketing campaigns by analyzing consumer sentiment across multiple platforms.
Conclusion
Data-driven demand forecasting is no longer optional for fashion brands and retailers looking to thrive. Intuition may spark creativity, but solid analytics point your brand toward profitable decisions and satisfied customers. By capturing insights from sales data, social media mentions, competitor benchmarks, and consumer sentiments, your organization can:
- Minimize Overstocks & Stockouts: Drive revenue, reduce markdowns, and design more efficient production cycles.
- Adapt Quicker to Emerging Trends: Launch collections that match market expectations before opportunities fade.
- Enhance Customer Loyalty: Provide the products consumers crave while demonstrating an understanding of shifting tastes.
Powerful platforms like Woven Insights bring these benefits within reach. From real-time consumer sentiment tracking to advanced modeling and interactive dashboards, Woven Insights equips fashion professionals with the tools to predict fashion demand effectively. The undisputed payoff lies in guided, data-backed decisions that deliver the right products at the right time—elevating brand perception and profitability on a global scale.
References
- Harvard Business Review: The Value of Customer Data and Analytics
- Intellias: Forecasting Demand and Sales in Fashion Retail
- Sqream: Big Data Helps Retail Revive
- Patents.Google.com: Anticipatory Shipping by Amazon
About Woven Insights
Woven Insights is a comprehensive market analytics solution that provides fashion brands with real-time access to retail market and consumer insights, sourced from over 70 million real shoppers and 20 million analyzed fashion products. Our platform helps brands track market trends, assess competitor performance, and refine product strategies with precision.
Woven Insights provides you with all the actionable data you need to create fashion products that are truly market-ready and consumer-aligned.
Click on the Book a demo button below to get started today.