Use Predictive Analytics to Reduce Stockouts and Waste
Discover how predictive analytics can transform your inventory management strategy by reducing stockouts and minimizing waste. Learn practical strategies and actionable insights to balance supply with demand using data-driven decision-making, with expert insights from WovenInsights.
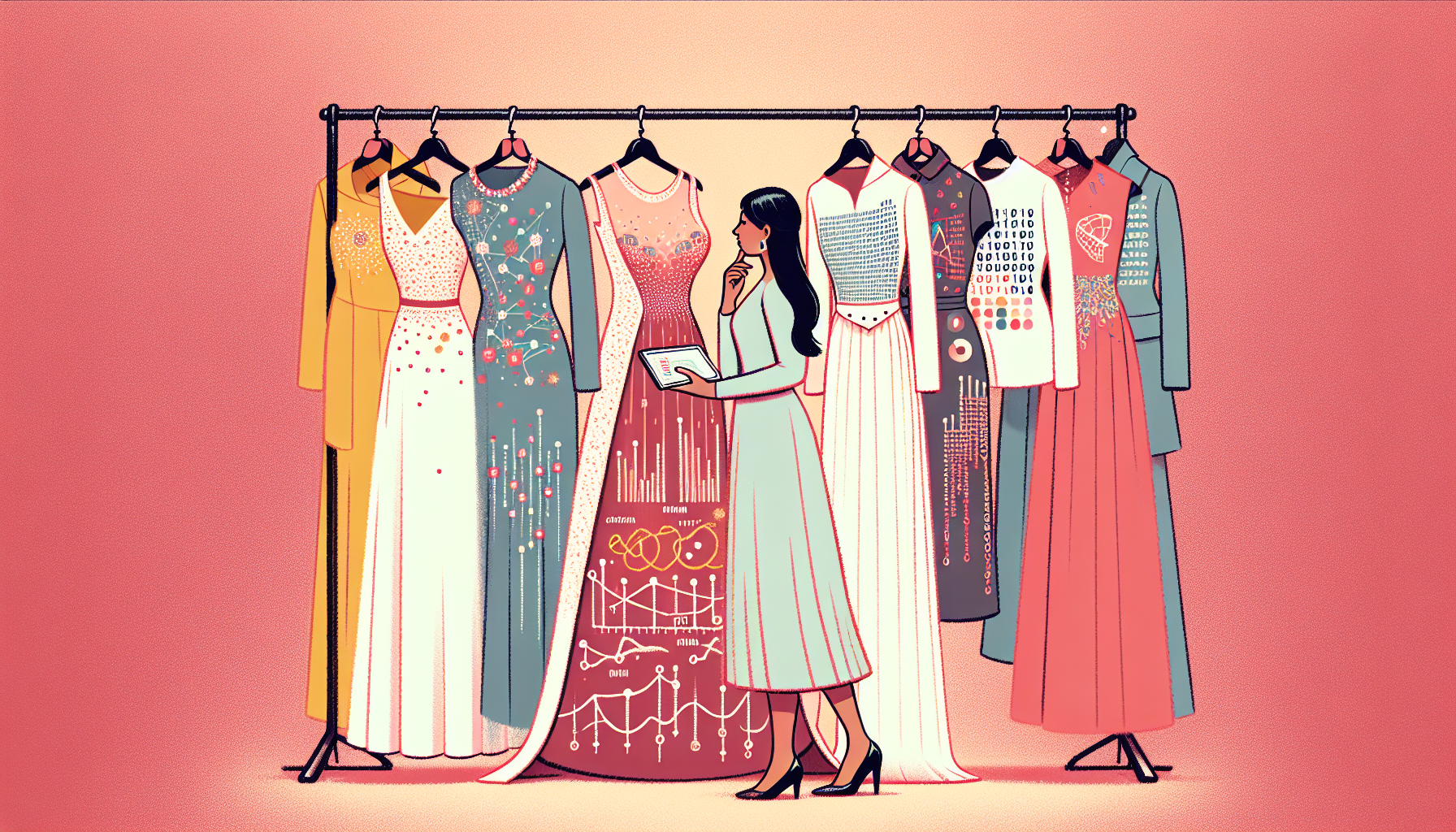
Table of Contents
- Introduction
- Understanding Stockouts and Inventory Waste
- The Role of Predictive Analytics in Inventory Management
- Key Strategies to Reduce Stockouts and Waste
- Case Study: Data-Driven Inventory Optimization
- How WovenInsights Can Empower Your Business
- Conclusion
Introduction
Inventory challenges, such as stockouts and excess waste, are a persistent concern for retail and e-commerce brands. Improving inventory management is critical for maintaining customer satisfaction and optimizing operational costs. This article explores how predictive analytics is a game-changer in addressing these challenges by enabling data-driven decision-making. With actionable insights and advanced forecasting techniques, businesses can not only reduce stockouts but also significantly cut down on waste.
In this discussion, we’ll look at practical strategies, supported by the capabilities of platforms like WovenInsights, to ensure inventory levels are optimized. This approach helps in balancing supply with demand and enhancing overall profitability.
Understanding Stockouts and Inventory Waste
Stockouts occur when inventory levels fall short of customer demand. This can lead to lost sales, diminished trust, and potential long-term negative impacts on brand reputation. Simultaneously, overstocking can result in increased waste and heightened carrying costs. Successfully managing this balance is vital to business success.
Key points to consider:
- Lost Revenue: Missing out on sales opportunities when customers turn to competitors.
- Waste Accumulation: Overstocking leads to unsold products, higher storage expenses, and potential product obsolescence.
- Operational Disruptions: Frequent stock adjustments can disrupt supply chain operations.
Achieving equilibrium between supply and demand not only enhances customer satisfaction but also drives cost efficiencies.
The Role of Predictive Analytics in Inventory Management
Predictive analytics harnesses historical data, external factors, and real-time information to create accurate forecasts. By analyzing patterns and trends, businesses can predict future demand with remarkable accuracy. This proactive approach enables brands to manage inventory levels more effectively, mitigating the risk of both stockouts and overstocking.
Analyzing Historical Data
Historical data analysis forms the backbone of predictive analytics. By examining past performance, trends, and customer behaviors, companies can:
- Detect Seasonality: Identify recurring patterns in sales data, such as holiday spikes or periodic slowdowns.
- Spot Trends: Understand how previous marketing campaigns, promotions, or external events influenced demand.
- Establish Baselines: Create benchmarks for expected inventory movement during various intervals.
Modern analytics platforms, like those offered by WovenInsights, make it simple to transform complex historical datasets into actionable insights. For example, a fashion retailer can analyze previous seasonal trends to ensure that upcoming collections are aligned with market demand, thereby preventing excess inventory and lost sales.
Incorporating Real-Time Data
While historical data is crucial, real-time analytics complete the picture. Integrating live data streams allows businesses to adjust forecasts as conditions change. This dual approach enables:
- Live Response to Changes: Quickly adapting to unexpected market shifts—be it due to competitor activities, sudden spikes in consumer demand, or supply chain disruptions.
- Dynamic Inventory Adjustments: Immediate reactions to changes in demand, ensuring that proactive measures are taken before potential stockouts occur.
- Enhanced Monitoring: Visualization dashboards that provide a real-time picture of inventory, helping teams make swift decisions.
By combining both historical and real-time data, predictive analytics empowers businesses to preemptively address inventory challenges. As a result, companies can maintain optimal stock levels, reducing waste and stockouts simultaneously.
Key Strategies to Reduce Stockouts and Waste
Implementing predictive analytics in inventory management involves various strategic initiatives. Here, we discuss actionable strategies for reducing stockouts and waste while enhancing overall efficiency.
Accurate Demand Forecasting
Accurate demand forecasting is the first step toward optimized inventory management. It involves:
- Leveraging Historical Sales Data: Understand patterns from past sales to predict future demand.
- Incorporating External Factors: Adjust forecasts based on market trends, seasonal effects, and promotional activities.
- Utilizing Machine Learning Models: Advanced models such as time series analysis help in capturing complex patterns, leading to more precise forecasts.
This data-driven method minimizes guesswork and ensures that inventory registers closely mirror consumer demand.
Optimizing Inventory Levels
Once demand projections are available, attention shifts to inventory optimization. Key practices include:
- Determining Optimal Stock Quantities: Calculate the ideal amount of inventory required to meet anticipated demand without incurring high carrying costs.
- Safety Stock Calculations: Establish safety stock levels to safeguard against uncertainties such as sudden demand spikes or supply delays.
- Continuous Inventory Monitoring: Regularly review turnover rates to ensure stock levels remain efficient.
These practices can significantly reduce the incidence of stockouts while also preventing waste from expired or obsolete stock.
Reducing Obsolete Inventory
Obsolete or slow-moving inventory not only ties up valuable capital but also leads to waste. Predictive analytics can help by:
- Identifying Slow-Moving Items: Use historical and real-time data to pinpoint products that are underperforming.
- Implementing Just-in-Time (JIT) Practices: Use predictive models to align inventory purchases with actual demand, which minimizes the need for excess stock.
- Monitoring Product Life Cycles: Adjust inventory levels in accordance with the product’s market life, thereby preventing overstocking of items nearing obsolescence.
Targeted actions such as timely promotions or discount strategies can further help in managing products moving slowly.
Enhancing Supplier Management
Strong supplier relationships are crucial for maintaining optimal inventory levels. Predictive analytics can improve supplier management by:
- Assessing Supplier Reliability: Analyze historical performance data of suppliers in terms of lead times and delivery accuracy.
- Optimizing Reorder Points: Utilize predictive insights to determine the best times and quantities for reordering, which reduces the risk of delays.
- Improving Supply Chain Transparency: Integrate real-time data to keep all stakeholders informed and responsive to potential disruptions.
This empathetic supplier management approach allows businesses to mitigate risks, ensuring a consistent supply of products.
Streamlining Operations
Operational efficiency is enhanced when data flows seamlessly between departments. Steps to improve operations include:
- Automating Replenishment Processes: Implement systems that automatically adjust order quantities based on predictive analytics insights, thus reducing manual errors.
- Integrating with ERP Systems: Synchronize inventory data with enterprise resource planning (ERP) systems for a holistic view of business operations.
- Regular Strategy Reviews: Periodically reassess inventory management policies in light of new data insights to maintain relevance and effectiveness.
Embracing automation and integration leads to a more responsive supply chain that quickly adapts to market dynamics.
Case Study: Data-Driven Inventory Optimization
Consider the case of an emerging fashion retailer struggling with periodic stockouts during peak seasons, causing customer complaints and revenue loss. The retailer turned to predictive analytics to revamp its inventory management strategy.
Challenge
The primary challenges were high variability in demand and a lack of clarity on which products were most affected by seasonal shifts. This led to frequent stockouts and, at times, over-ordering, which increased waste.
Implementation
By leveraging a robust predictive analytics platform, the retailer took the following steps:
- Historical Data Analysis: Mapped out sales data over the previous two years to identify demand patterns and seasonal trends.
- Integration of Real-Time Data: Established live dashboards to track current inventory and sales metrics, enabling immediate response to deviations.
- Machine Learning Models: Applied time series analysis and regression models to forecast demand more accurately.
- Strategic Replenishment: Adjusted their reorder points and safety stock levels based on these predictive insights.
Results
- Reduced Stockouts: The retailer experienced a significant decrease in stockouts during high-demand periods, leading to increased customer satisfaction.
- Optimized Inventory Levels: Over-ordering was curtailed, reducing stored waste and associated costs.
- Operational Efficiency: The overall supply chain became more agile, capable of quickly adapting to unexpected market changes.
This real-world example underscores how predictive analytics transforms inventory management from reactive to proactive.
How WovenInsights Can Empower Your Business
Platforms like WovenInsights take the guesswork out of inventory management. Here’s how its features align with the strategies discussed:
- Customer Sentiment Analysis: Beyond numbers, understand customer feedback to gauge potential demand shifts. Satisfied customers tend to translate into predictable buying patterns.
- Market Trend Analysis: Stay ahead by monitoring market trends and consumer behaviors, ensuring your product offerings are continuously in sync with evolving tastes.
- Competitor Benchmarking: By comparing your performance with industry leaders, you can refine inventory strategies and ensure competitive pricing.
- Retail Performance Metrics & AI-Powered Recommendations: Use intelligent forecasting and real-time data to adjust reorder points and safety stock levels dynamically.
WovenInsights not only provides these robust analytics but also offers user-friendly dashboards that simplify the interpretation of complex data, making it an essential tool for modern retail and e-commerce operations. For more details on harnessing the power of data-driven decisions, visit the WovenInsights website.
Conclusion
Predictive analytics is revolutionizing the way retail and e-commerce brands manage inventory. By accurately forecasting demand, reducing the risk of stockouts, and minimizing waste, businesses can optimize their operations and improve profitability.
Adopting strategies such as leveraging historical data, incorporating real-time updates, fine-tuning inventory levels, and enhancing supplier performance not only increases efficiency but also drives better customer satisfaction. The integration of powerful analytics platforms like WovenInsights ensures that your business stays agile in the face of market variability.
Ultimately, embracing predictive analytics is about making smarter, data-driven decisions that balance supply and demand effectively. As you implement these insights, you’ll find that reducing stockouts and minimizing waste becomes not only achievable, but a catalyst for sustainable growth and operational excellence.
By continuously refining your approach and investing in advanced analytics, your retail or e-commerce brand can secure a competitive edge in today’s fast-paced market. Embrace the future of inventory management, and start leveraging the full power of predictive analytics to transform your business.